In in the present day’s international panorama, organizations worldwide are more and more turning to knowledge analytics to reinforce their enterprise efficiency. Analysis carried out by McKinsey Consulting revealed that data-driven corporations not solely expertise above-market development but additionally witness EBITDA (Earnings Earlier than Curiosity, Taxes, Depreciation, and Amortization) will increase of as much as 25% [1]. Moreover, Forrester’s findings point out that organizations using knowledge to derive insights for decision-making are almost 3 times extra prone to obtain double-digit development [2].
Basically, the cornerstone of deriving actionable insights from knowledge lies within the growth of sturdy analytics fashions, which function the essential bridge between uncooked knowledge and useful insights. However what precisely constitutes an analytics mannequin? The time period “analytics fashions” is often employed within the realm of analytics, but it’s susceptible to misuse and misinterpretation attributable to its reliance on the meant function, obtainable sources, and different constraints. The time period “analytics fashions” has certainly develop into considerably of a cliché, typically overused, and missing in authentic thought.
Technically, an analytics mannequin serves as a mathematical illustration of a real-world system, facilitating insights into its conduct. It organizes enterprise classes, entities, and occasions by using knowledge variables and frameworks, using equations or algorithms. The first goal of such a mannequin is to derive insights that help decision-making and the implementation of acceptable enterprise methods, leveraging knowledge because the enter. A high-quality analytics mannequin reveals three essential traits:
- Generic: A sturdy analytics mannequin not solely performs nicely on the information it was initially skilled on but additionally produces dependable insights when utilized to new knowledge inside the present enterprise mannequin. It ought to seize the important system traits whereas abstracting away pointless particulars.
- Sturdy: The analytics mannequin ought to possess adaptability, enabling it to replicate modifications within the knowledge, notably in relation to evolving enterprise fashions.
- Scalable: Efficient analytics fashions ought to display the aptitude to swiftly course of and analyze massive volumes of various knowledge, catering to each present and new enterprise fashions.
Basically, there exist quite a few approaches to formulating analytics fashions, and broadly talking, analytics fashions might be conceptualized from 4 main views or manifestations, as illustrated in Determine 1 under.
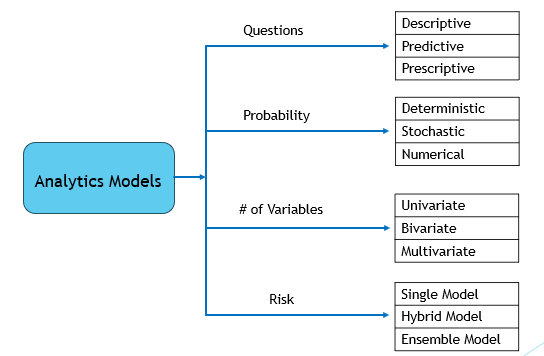
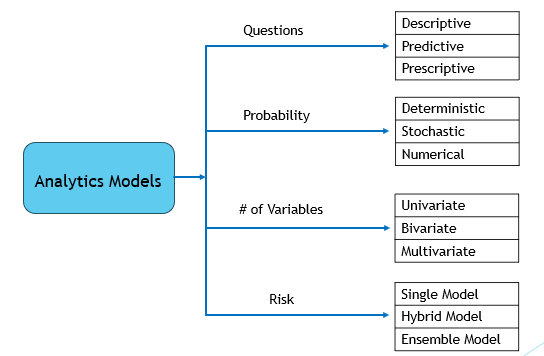
Analytics, essentially, entails questioning to extract insights from knowledge for the aim of measuring and enhancing enterprise outcomes [3]. Relying on the character of those inquiries, three kinds of analytics fashions emerge:
- Descriptive analytics fashions: These fashions tackle the query, “What occurred?” Descriptive analytics delves into historic knowledge to discern previous patterns, traits, and relationships. It employs exploratory, associative, and inferential knowledge evaluation methods. Exploratory knowledge analytics fashions scrutinize and summarize datasets, whereas associative descriptive analytics fashions elucidate the relationships between variables. Inferential descriptive knowledge evaluation is utilized to deduce or extrapolate traits a few bigger inhabitants based mostly on a pattern dataset.
- Predictive analytics fashions: These fashions give attention to answering the query, “What’s going to occur?” Predictive analytics entails the method of using knowledge to forecast future traits and occasions. Predictive evaluation can both be carried out manually, generally known as analyst-driven predictive analytics, or via the utilization of machine studying algorithms, also referred to as data-driven predictive analytics. In both case, historic knowledge serves as the idea for making future predictions.
- Prescriptive analytics fashions: These fashions help in answering the query, “How can we make it occur?” Basically, prescriptive analytics recommends the optimum plan of action for development, using optimization and simulation methods. Usually, predictive evaluation and prescriptive analytics are intertwined, as predictive analytics identifies potential outcomes whereas prescriptive analytics explores these outcomes and identifies additional choices.
One other perspective on analytics fashions is predicated on the extent of uncertainty or chance related to deriving insights. This classification yields three kinds of analytics fashions:
- Deterministic fashions: These fashions present insights precisely with certainty. Usually, insights derived from historic knowledge fall into this class.
- Stochastic fashions: These fashions incorporate randomness or uncertainty into the mannequin. Insights relating to future states derived from Regression and Gradient Boosting Machines (GBM) fashions exhibit a degree of uncertainty and are thought of stochastic.
- Numerical fashions: These fashions are utilized to approximate the conduct of complicated programs by dividing them into smaller, extra manageable parts, that are then solved iteratively utilizing computational strategies. Examples of numerical fashions embody the Finite Ingredient Technique (FEM) utilized in structural engineering, the Climate Analysis and Forecasting (WRF) mannequin employed in climate prediction, and epidemiological fashions used to simulate the unfold of illnesses.
Analytics fashions might be categorized based mostly on the variety of variables within the mannequin. On this regard, the information analytics fashions might be univariate, bivariate, or multivariate [4].
- Univariate analytics fashions: Univariate evaluation entails analyzing the sample current in a single variable utilizing measures of centrality (imply, median, mode, and so forth) and variation (commonplace deviation, commonplace error, vary, variance, and so forth).
- Bivariate analytics fashions: There are two variables whereby the evaluation is said to trigger and the connection between the 2 variables. These two variables might be dependent or unbiased of one another. The correlation method is probably the most used bivariate evaluation method.
- Multivariate analytics fashions: This mannequin is used for analyzing greater than two variables. Generally used multi-variate analytics fashions are Linear Regression, Logistic Regression, and Assist Vector Machines.
Lastly, no matter the kind of mannequin chosen, managing related dangers is essential in analytics. From a threat perspective, analytics fashions might be categorized into three principal sorts:
- Single fashions: These fashions make the most of a single algorithm to derive insights. Examples embody linear regression, determination bushes, logistic regression, and assist vector machines, amongst others. Single fashions are easy to implement and usually carry out adequately for the given dataset and drawback.
- Hybrid fashions: Hybrid fashions mix two totally different analytics fashions. For example, a hybrid classification mannequin may consist of 1 unsupervised learner (or cluster) for preprocessing the coaching knowledge and one supervised learner (or classifier) for studying the clustering outcome, or vice versa.
- Ensemble fashions: Ensemble fashions make use of quite a lot of totally different algorithms on the identical or totally different datasets to supply outputs. The amalgamation of fashions typically yields superior efficiency in comparison with utilizing a single mannequin or a hybrid mannequin. Basically, ensemble fashions contain a number of fashions working independently of one another to generate insights utilizing a voting system.
Knowledge analytics is revolutionizing enterprise fashions by fostering the creation of recent income streams, driving expense discount, and mitigating dangers for enterprises throughout various business sectors. The importance of choosing the suitable analytics mannequin can’t be overstated, because it performs a pivotal position in enabling corporations to appreciate the specified enterprise influence. The 4 manifestations of knowledge analytics fashions outlined right here provide a framework for successfully deploying analytics tailor-made to particular enterprise necessities, present capabilities, and useful resource availability. Consequently, leveraging these fashions enhances the chance of delivering profitable analytics initiatives, thereby fostering improved enterprise outcomes.
References
- McKinsey, “Insights to influence: Creating and sustaining data-driven business development,” January 2022
- Evelson, Boris, “Insights Investments Produce Tangible Advantages — Sure, They Do,” Might 2020
- Southekal, Prashanth, “Analytics Finest Practices,” Technics, 2020