Lendbuzz blends its founders’ early credit score experiences with synthetic intelligence (AI) to disrupt conventional evaluation strategies and widen the pool of credit-worthy people. And with the founding crew’s deep understanding of expertise, they capitalized on the correct applied sciences on the proper time.
Co-founder and CEO Amitay Kalmar mentioned the seeds for Lendbuzz had been planted throughout his and co-founder Dan Raviv’s early experiences in the USA. Regardless of promising instructional paths at high universities and good account balances, they struggled to get auto loans and primary bank cards. Household and buddies shared related tales.
The pair understood various information, machine studying and deep neural networks nicely. They knew they might be used to evaluate creditworthiness higher and assist underserved teams.
Kalmar and Raviv started by specializing in software program options for auto loans, prioritizing people with no credit score file. However they quickly noticed that their system was higher at scoring candidates in lots of areas. That was excellent news to the 45 million People who both had no credit score file or one which was too skinny to be successfully scored by FICO.
“Inside these tens of tens of millions of individuals, clearly, they’re not all unhealthy shoppers,” Kalmar mentioned. “They’re not all not creditworthy. It’s simply there is no such thing as a system to guage them.”
Lendbuzz deployed the correct expertise on the proper time
Timing is a vital facet of Lendbuzz’s story. Digital methods and cloud expertise have matured sufficient to be helpful for fintechs. Digital information and APIs abound. Storage and computational capability have grown. Knowledge fashions can now run hundreds of knowledge factors. These advantages didn’t exist a decade in the past.
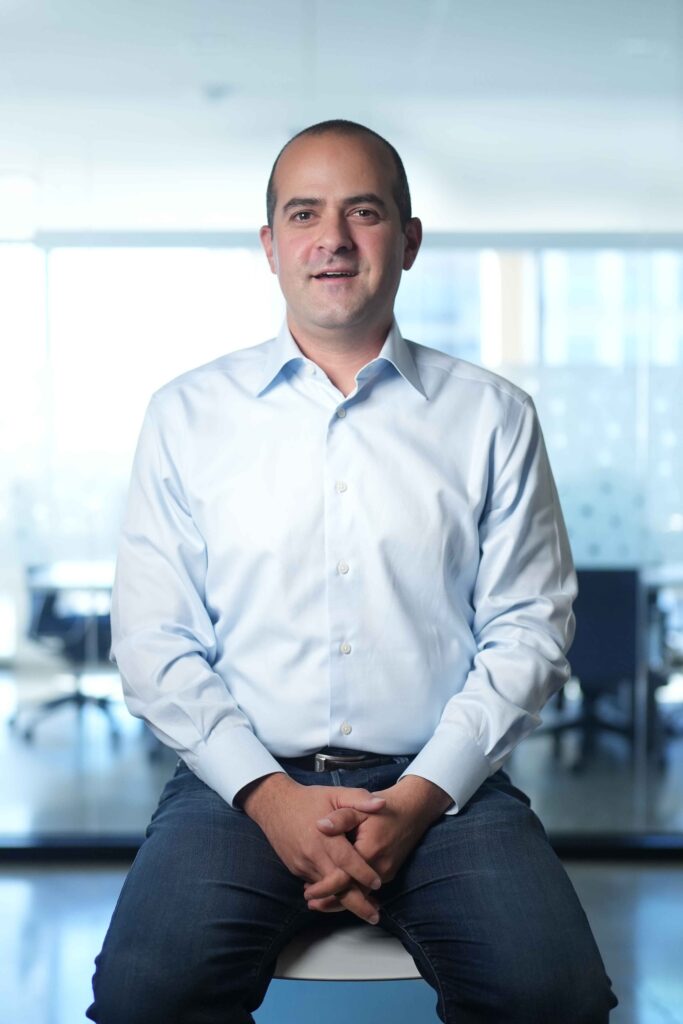
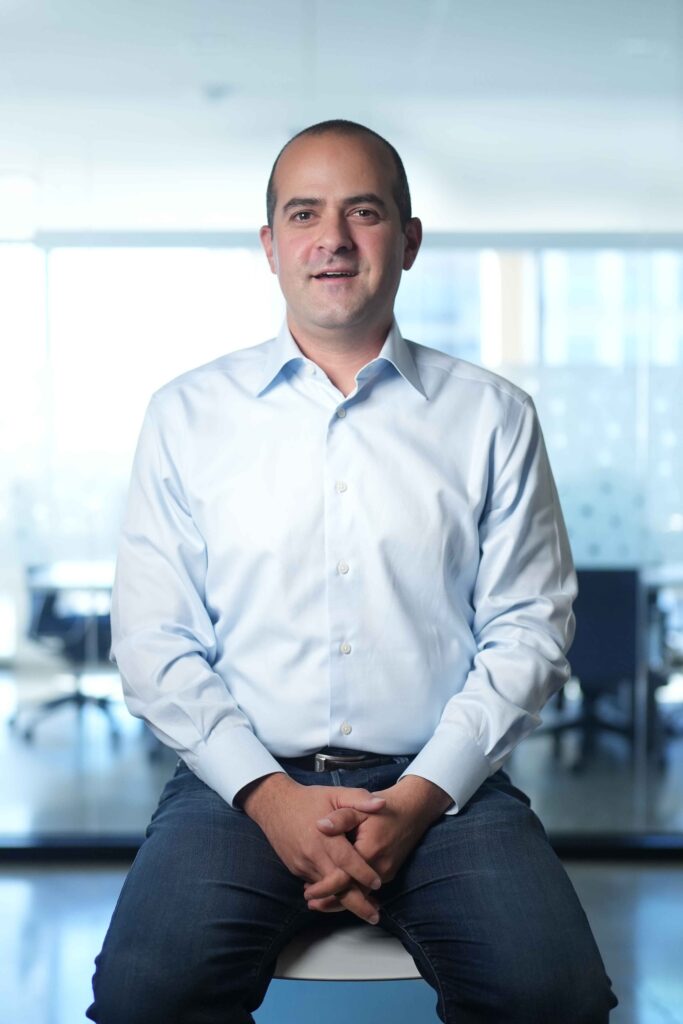
“You may have the instruments to research hundreds of knowledge factors on a person and run tens of tens of millions of fashions to enhance your modelling,” Kalmar mentioned. “So why not use it? Why nonetheless be caught with a credit score file that has minimal information units and serves a really particular shopper however excludes many others?”
Round 2015, Kalmar and Raviv started analyzing Lending Membership loans and noticed arbitrage alternatives. However to maximise these openings, they wanted distinctive information units. From their analysis, Kalmar and Raviv noticed that augmented information enhanced credit score assessments.
That was the place they started to view the issue otherwise by taking a look at incremental information enhanced by machine studying and deep neural community algorithms via a localized system. That enables methods to contemplate every shopper as a vector that’s higher understood as extra alerts are collected.
Embracing various information and why the US lags behind
Lendbuzz expertise is partially formed by Raviv’s experiences finding out how a Mexican financial institution thought of various information sources. Kalmar mentioned there may be a lot to study from how a lot of the world crunches various information.
The USA is late to that get together as a result of it’s among the many finest in accumulating credit score bureau information. Few international locations have such an developed system.
“FICO, on the finish of the day, works rather well,” Kalmar mentioned. “Should you had a 750 FICO and a deep file, you’re unlikely to default. When you have a 450 FICO and some delinquencies, you’ll possible default, creating a very good separation. The truth that the info is so good and deep right here, and there are three credit score bureaus, and each fee is collected, is stopping innovation in underwriting.
“So if you happen to’re in an rising nation, Asia, Latin America, or Africa, you don’t have that system. Whether or not you’re a small or massive establishment, you need to do higher in your underwriting; it is advisable innovate and do one thing completely different. It’s essential use a unique information set.”
Disruption is tougher as you develop
It’s additionally a lot simpler to innovate from the start than after you’re established. Kalmar sees that as Lendbuzz matures and appreciates how arduous it’s at a legacy establishment. Some adjustments might also value greater than they profit the corporate.
“Disruption normally comes from new entrants to an area,” Kalmar mentioned. “I don’t suppose it’s very completely different than shopper credit score. There are occasions when disruption comes from incumbents, and it’s very admirable as a result of it’s a lot tougher to do.”
How Lendbuzz meets a triangle of wants
Kalmar mentioned Lendbuzz’s system is exclusive as a result of it has three stakeholders: debtors, lenders and sellers. Their system should ship worth to extra sides. The software program focuses on offering sellers with a streamlined mortgage software that simplifies origination. The machine learning-based system crunches 18 months of financial institution information inside seconds of connecting an applicant’s financial institution data. That gives essential insights on earnings, spending patterns, training, employment, and even automobile utilization.
“The hundreds of options we acquire on every particular person go into deep neural networks, after which we use a localized system to attain the person,” Kalmar defined. “That creates a proprietary danger rating, which we use to make a credit score choice on whether or not this particular person matches our credit score profile or doesn’t.
“Each little factor is a characteristic. Then you might have that profile of a person, and now you solely care about in the event that they make a fee or not. Each month, you acquire new alerts on that particular person. You evaluate that new profile to probably the most related 500 different shoppers that we originated within the final three years.”
Validation, thy identify is a $219M AAA securitization
The proof of Lendbuzz’s success is in a really profitable pudding. Earlier this month, Lendbuzz accomplished a $219 million securitization collateralized by a pool of auto loans. The transaction was rated AAA. Three years in the past, the corporate’s first securitization was A-. That’s some validation.
“Our efficiency over time confirmed them that we considerably outperformed the market in a really difficult cycle of shopper credit score,” Kalmar mentioned. “The previous 24 months have been a difficult cycle, and shopper credit score delinquencies have shot via the roof, particularly for non-prime shoppers.
“We’ve been very constant in our efficiency, performing higher than the credit score agencies-based fashions, whereas most conventional lenders have carried out a lot worse than the bottom fashions. So over time, we had been capable of improve our credit standing.”
AI’s disruptive potential is generational
Lendbuzz isn’t sitting on its laurels. Kalmar mentioned he’s watching generative AI, which he believes might have as a lot influence because the Web, although it might take as much as 20 years for it to be fulfilled. Early adopters will profit probably the most, although that comes with a caveat.
Kalmar mentioned it’s attainable to behave too early. Key infrastructure items aren’t in place. Wager on the unsuitable pipes, and also you miss the boat. Carefully watch developments, have a crew in place and be able to pounce.
“We’re not going to construct every little thing in generative AI,” he mentioned. “We have to construct on infrastructure like we’re constructing on Amazon cloud infrastructure. The truth that we are able to use it permits us to do issues we couldn’t have performed 10 years in the past.
“The identical factor with generative AI. So we’re trying deep into the infrastructure there. What will we need to base our options on? All of our time and new R&D can be centered on these areas. Massive alternative.”
Additionally learn: